ECCV 2020 Virtual Tutorial on
New Frontiers for Learning with Limited Labels or Data
Learning with limited data or labels remains an important unsolved problem in computer vision. It prevents us from democratizing AI technology today. While humans accomplish this task seamlessly, contemporary AI algorithms struggle with it. So, how far along have we come towards solving this problem in vision? What are some new frontiers to move forward? In this tutorial we will explore several emerging ideas and exciting breakthroughs that are pushing the boundaries of what is possible for this task. We will first describe exciting new accomplishments in self-supervised learning from image collections and videos, which are helping to learn a myriad of vision tasks. Next we will show emerging ideas around learning with imperfect labels and of unification across different levels of supervision. Lastly, we will present two new ideas around inverting networks to provide data or feedback, which can help to learn in limited data scenarios.
Speakers
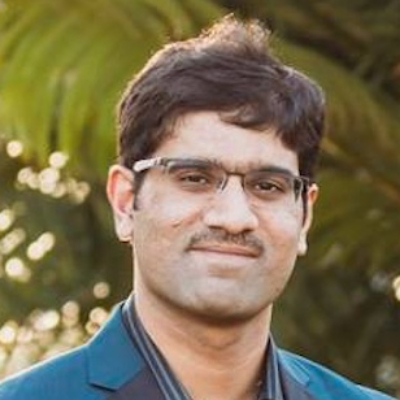
Google Research
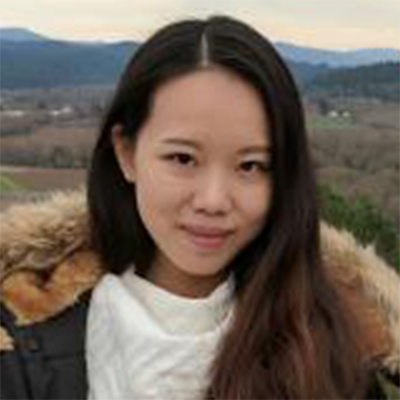
NVIDIA
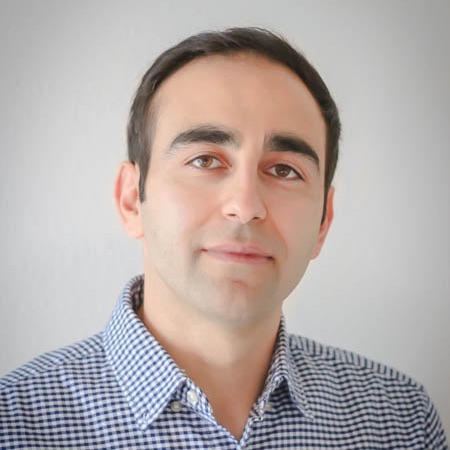
NVIDIA
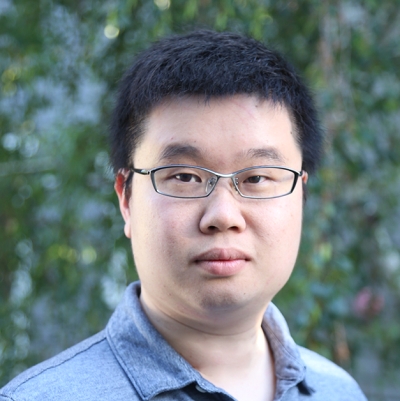
NVIDIA
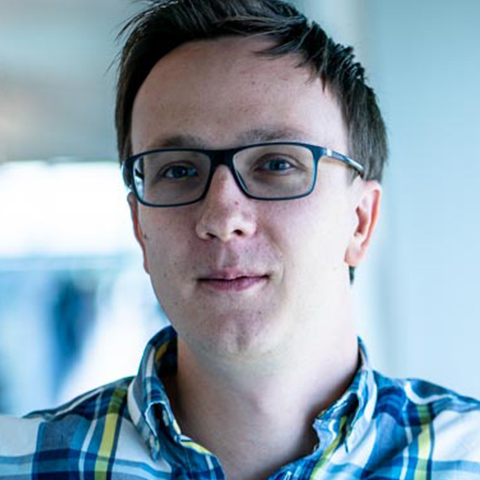
NVIDIA
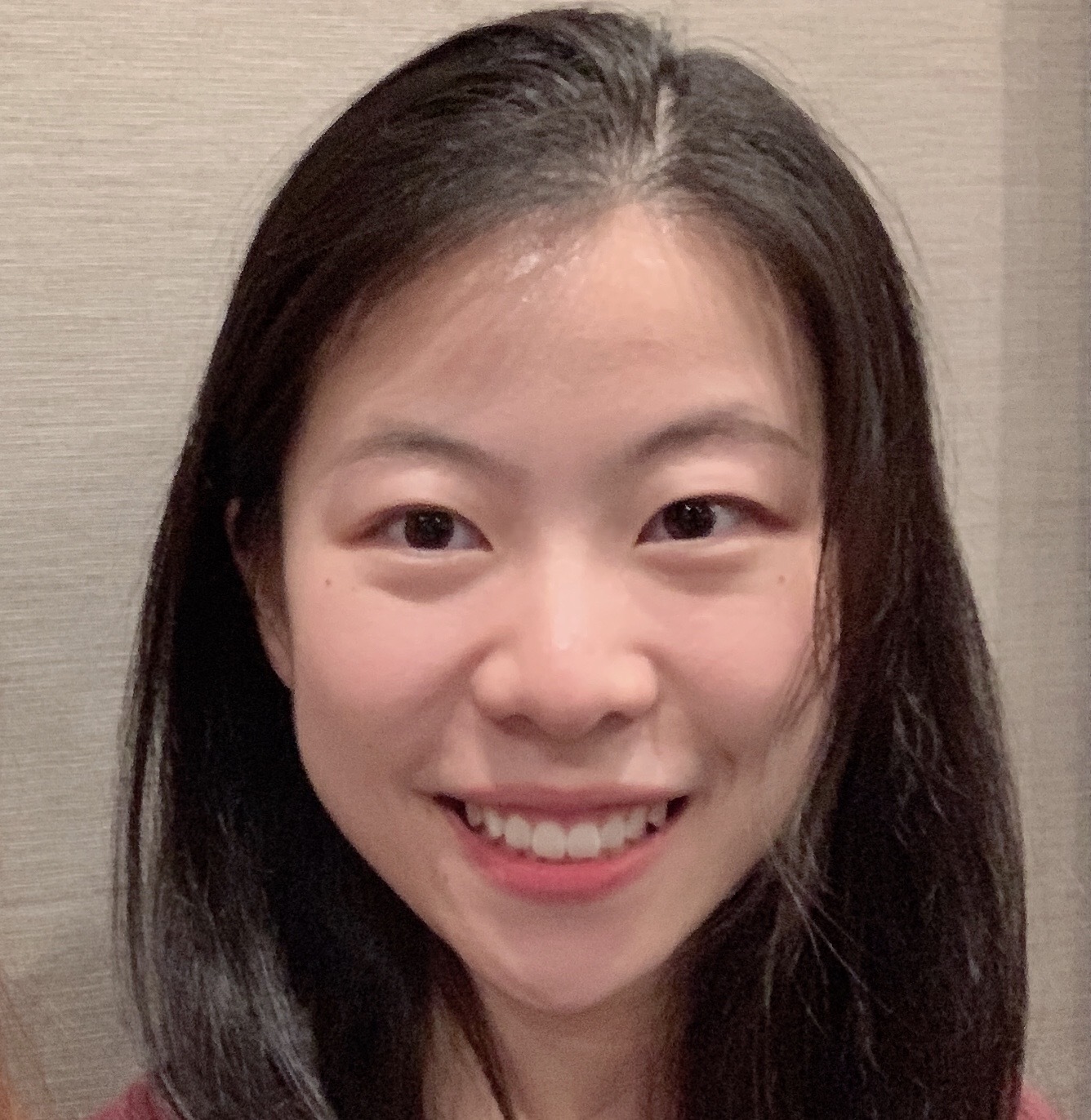
Caltech
Talks
Title | Speaker | Live Session | ||
---|---|---|---|---|
Introduction | Shalini De Mello | Video | ||
Self-supervised Learning | ||||
Self-Supervised Part and Viewpoint Discovery from Image Collections | Varun Jampani | Video | ||
Learning Visual Correspondences across Instances and Video Frames | Sifei Liu | Video | ||
Imperfect Labels | ||||
Limitless Labels in a Labelless World: Weak Supervision with Noisy Labels | Arash Vahdat | Video | ||
Learning with Imperfect Labels and Visual Data | Zhiding Yu | Video | ||
Inverting Neural Networks | ||||
Inverting Neural Networks for Data-free Knowledge Transfer | Pavlo Molchanov | Video | ||
Learning Efficiently with Biologically Inspired Feedback | Yujia Huang | Video |
Organizers
Shalini De Mello is a Principal Research Scientist in the Learning and Percpetion Research group, NVIDIA. Her research interests are in computer vision, machine learning, learning with limited data or labels (supervised-supervised, few-shot and with synthetic data) and human-computer interaction (gaze and head pose estimation). Her research has lead to the development of NVIDIA’s innovative DriveIX product for smart AI-based automotive interfaces. She received doctoral and master’s degrees in Electrical and Computer Engineering from the University of Texas at Austin in 2008 and 2004, respectively. She received the outstanding reviewer award for ICCV 2019, and won first place in the OpenEDS 2019 and Hands 2015 challenges.
Sifei Liu is a Senior Research Scientist in Learning and Perception Research group, NVIDIA. She obtained her Ph.D. at the University of California Merced, Department of EECS, advised by Professor Ming-Hsuan Yang. Her research interests are in computer vision (low-level vision, semantic segmentation and 3D scene understanding), deep learning (graph-structured modules, self-supervised learning), and the combination of both. She worked as an intern student in Baidu IDL from 2013 to 2014, multimedia lab in CUHK in 2015, and NVIDIA research in 2017. She is also the recipient of the Baidu Graduate Fellowship in 2013 and the Rising Star EECS in 2019. She has co-organized the Learning Representations via Graph-structured Networks tutorial in conjunction with CVPR 2019 and 2020.
Zhiding Yu is a Senior Research Scientist in the Machine Learning Research group, NVIDIA. He obtained a Ph.D. in ECE from Carnegie Mellon University in 2017 and an M.Phil. in ECE from The Hong Kong University of Science and Technology in 2012. His research interests include deep representation learning, weakly/self-supervised learning, transfer learning and deep structured prediction, along with their applications to vision and robotics problems. He conducted internships at Adobe Research, Microsoft Research and Mitsubishi Electric Research Laboratories in 2013, 2015 and 2016. He was twice the recipient of the HKTIIT Post-Graduate Excellence Scholarships in 2010 and 2012. He is a co-author of the best student paper at ISCSLP 2014, an author of the best paper award at WACV 2015, and a winner of the WAD Challenge 2018. His work on deep facial expression recognition at Microsoft Research won first runner-up at the EmotiW-SFEW Challenge 2015 and was integrated into the Emotion Recognition API under Microsoft Azure Cognitive Services.
Pavlo Molchanov is a Principal Research Scientist at Learning and Perception Research team, NVIDIA. He obtained his PhD from the Tampere University of Technology, Finland in the area of signal processing in 2014. His dissertation was focused on designing automatic target recognition systems for radars. His research is focused on methods for neural network acceleration, and designing novel systems for human-computer interaction and human understanding. In the area of network acceleration, he is interested in neural network pruning methods and conditional inference. For human understanding, he is working on landmark estimation, gesture recognition, hand pose estimation. He received the EuRAD best paper award in 2011 and EuRAD young engineer award in 2013.
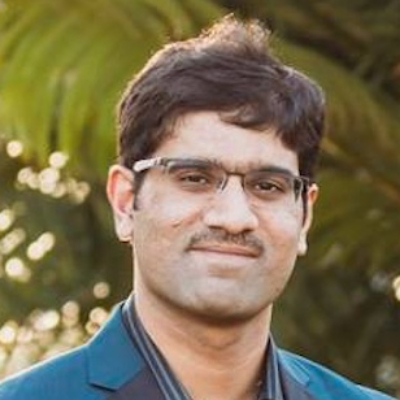
Google Research
Varun Jampani is a Research Scientist at Google Research in Cambridge, US. Prior to that, he was a Research Scientist at NVIDIA. He works in the areas of machine learning and computer vision and his main research interests include content-adaptive neural networks, self-supervised visual discovery and novel view synthesis. He obtained his PhD with highest honors at Max Planck Institute for Intelligent Systems (MPI) and the University of Tübingen in Tübingen, Germany. He obtained his BTech and MS from the International Institute of Information Technology, Hyderabad (IIIT-H), India, where he was a gold medalist. He was an outstanding reviewer at CVPR'19, CVPR'18, CVPR'17 and ECCV'16. His work on 'SplatNet' has received 'Best Paper Honorable Mention' award at CVPR'18.
Arash Vahdat is a Senior Research Scientist at NVIDIA specializing in machine learning and computer vision. Before joining NVIDIA, he was a research scientist at D-Wave Systems where he worked on weakly supervised learning from noisy labeled data and deep generative learning with discrete latent variable models. Prior to D-Wave, Arash was a Ph.D. student under Greg Mori’s supervision working on latent variable frameworks for visual analysis, then a research faculty member at Simon Fraser University, where he led several research projects at the intersection of deep learning and video analysis. His area of research includes generative learning, weakly-supervised learning, neural architecture search, and probabilistic deep learning.
Yujia Huang is a PhD candidate in Electrical Engineering at Caltech, advised by Prof Anima Anandkumar. She obtained her bachelor’s degree from Zhejiang University, China in 2017. Her research interests are in generative models, uncertainty quantification and biologically inspired machine learning, with an emphasis on vision tasks. She is an intern in the Machine Learning Research gourp Research, NVIDIA during the summer of 2020.
Anima Anandkumar is the Director of Machine Learning Research at NVIDIA and Bren Professor at Caltech. She was previously a Principal Scientist at Amazon Web Services. She has received several honors such as Alfred. P. Sloan Fellowship, NSF Career Award, Young investigator awards from DoD, and Faculty Fellowships from Microsoft, Google and Adobe. She is part of the World Economic Forum's Expert Network. She is passionate about designing principled AI algorithms and applying them in interdisciplinary applications. Her research interests include tensor methods, optimization, and large-scale learning.
Jan Kautz is Vice President of Learning and Perception Research at NVIDIA. Jan and his team pursue fundamental research in the areas of computer vision and deep learning, including visual perception, geometric vision, generative models, and efficient deep learning. Before joining NVIDIA in 2013, Jan was a tenured faculty member at University College London. He holds a BSc in Computer Science from the University of Erlangen-Nürnberg (1999), an MMath from the University of Waterloo (1999), received his PhD from the Max-Planck-Institut für Informatik (2003), and worked as a post-doctoral researcher at the Massachusetts Institute of Technology (2003-2006).